No, AI Queries & Images Aren’t Carbon Bombs, So Stop Hyperventilating
Regular readers will either appreciate or hate that for roughly 20 months I’ve been decorating my articles and presentations with images generated by artificial intelligence algorithms. I’m not going to address the full range of reasons, but will just do some basic math on the electricity use to get a subset of regular readers to give up already.
This isn’t the first time I’ve dipped my toes into these waters, of course. Five years ago, I did a global assessment of Cloud computing service providers to assess that round of “compute power is killing us!” hysteria. I wouldn’t have recommended Alibaba at the time, but other major providers were buying green electricity with PPAs and buying high-quality carbon credits.
Later that year, I had to return to the subject because one of the first “training our future AI overlords is killing us!” hype cycles was underway. I took apart the weak assumptions of the MIT study that found that, and returned to ignoring the hysteria.
I won’t point fingers, but even CleanTechnica writers who should know better have been quoting people who clearly don’t know what they are talking about this year, part of the current “okay, ChatGPT is really useful but it’s killing us!” hype cycle. To be blunt, anyone who has ever written about Tesla’s AI-powered autonomous driving features should never have fallen for this, but people generally don’t like doing math.
So let’s disambiguate a bit. It’s not rocket science.
First, large language models (LLMs) and generative image models (GIMs) do require a lot of electricity, but only to train them. Enormous amounts of data is assembled. An approach to ingesting that data is used. It occurs. That ingestion and processing is very energy intensive. Training the current OpenAI ChatGPT 4o model is reported to have required 10 gigawatt-hours. That’s not a remotely trivial amount of energy. DALL-E 3.0 probably required 1 to 2 GWh.
But querying the models doesn’t require enormous amounts of electricity, about 0.001-0.01 kWh per query. In computer science, there’s a rule of thumb that if it’s fast to put something into storage, it’s slower to take it out, and vice versa. Part of the reason LLMs and GIMs take a lot of time to process is because they are being optimized for fast responses. The intent is to amortize that 1-10 GWh over potentially billions of queries.
Let’s pretend that OpenAI’s California team and other almost entirely coast-based, liberal elite, climate-aware developers of LLMs and GIMs are complete idiots. Let’s pretend that they use US grid average electricity, about 0.4 kg CO2e per kWh for generating their LLMs and GIMs. How much carbon debt would accrue?
A gigawatt-hour would be 400 tons of CO2e. 10 GWh would be 4,000 tons. That’s like the carbon debt of 270 to 2,700 Americans’ average driving. It would be a reasonable amount, but it’s a small town’s worth of annual driving (which should be a hint about the real problem in America).
But they aren’t complete idiots, as I pointed out in 2019 a couple of times. They know the carbon debt of electricity and aren’t coal barons. Taking OpenAI as an example, it does all of its computing on Microsoft Azure’s cloud platform, the one I ranked most highly in 2019 for low carbon concerns.
Microsoft buys renewable electricity for a lot of its data centers, and is currently at 44% of annual electricity supplied by power purchase agreements for wind and solar. Further, it puts its data centers next to hydroelectricity whenever possible to soak up low carbon hydro kWh.
So let’s assume that OpenAI and Microsoft were still pretty dim, and positioned all of that computing in an Azure data center that’s only 56% better than average, or 0.22 kg CO2e per kWh. That 400 to 4,000 tons shrinks to 220 to 2,200 tons of CO2e, 150 to 1,500 American drivers’ worth to train the models.
However, OpenAI is based in California in the San Francisco region, California’s grid has slimmed down to 0.24 kg CO2e per kWh and Microsoft is buying renewable electricity for SF-area data centers too. 56% of 0.24 kg CO2e is 0.13 kg CO2e / kWh. At that carbon intensity, training the models produces 130 to 1,300 tons of CO2e. Is this something to write home about joyously? No, but we are down to 90 to 900 American drivers, a village worth of people.
But let’s ask the next question. This is the slow in part of the data manipulation process, not that fast retrieval process. As such, it needs to be amortized across the number of ChatGPT queries or DALL-E images generated. Let’s be reasonably fair and assume that the models only last six months before being replaced, so the carbon debt is only spread over six months of queries and images.
How many ChatGPT queries are there a month? There are 1.8 billion visits a month, and they last about 7 to 8 minutes per the data I was able to find. That suggests 3-6 queries per visit. Let’s assume 4 queries, so that’s about 7 billion queries a month and about 43 billion queries for the life of the model. That 1,300 tons of CO2e is divided by 43 billion to get the carbon debt per query, or about 0.03 grams per query.
By contrast, DALL-E, which has a lower carbon debt, generates about two million images a day, or about 365 million images in half a year. That’s about 0.356 grams per image. Wow, three of those and you would be over a gram of CO2e.
Oh, but wait, we aren’t finished. Now we have to actually run a query or generate an image. Remember how much energy that takes, 0.001-0.01 kWh per query. At 0.4 kg per kWh, that’s 0.4 to 4 grams per query. But remember, OpenAI runs its services on Microsoft Azure, and Microsoft is buying GWh of renewable electricity and tons of high quality carbon credits (unlike a lot of the breed).
Let’s take the US average at 56%. That’s 0.2 to 2.2 grams CO2e per query or image. California’s would be 0.07 to 0.7 grams.
Let’s take the Azure data center closest to me that isn’t dedicated to Canadian confidential information, and hence the one by far most likely to be running my queries and generating my images. It’s in Quincy, Washington, which is strategically situated near several major hydroelectric facilities. Just 85 miles north lies the Grand Coulee Dam, with a capacity of over 6,800 megawatts. The Chief Joseph Dam, located about 50 miles north, contributes 2,614 megawatts of power. Wells Dam, approximately 70 miles north, operated by Douglas County PUD, provides 840 megawatts of renewable energy. Closer to Quincy, about 40 miles west, is the Rocky Reach Dam, offering 1,300 megawatts, and the Rock Island Dam, 30 miles west, adds another 624 megawatts.
What is the Quincy Azure cloud data center’s likely CO2e per kWh? Probably around 0.019 kg CO2e/kWh. The carbon intensity of my average query or image is around 0.000019 grams of CO2e. Add in the 0.365 grams of carbon debt per image and I’m still at 0.365 grams. Add in the 0.03 grams per ChatGPT query and I’m still at 0.03 grams. Gee, let me go buy some high-quality carbon credits to cover that. Oh, wait. The average cup of coffee has a carbon debt of 21 grams, dozens or hundreds of times higher? And I’d have to create four billion images to equal a single American driver’s carbon habit? Never mind.
Oh, wait, haven’t I debunked this enough? You’re complaining that I’m only counting compute, not air conditioning? Well, guess what, modern data centers run at 1.1 power usage effectiveness. That means that for every unit of electric, they use 10% extra for power, lights, and the like. Go ahead, add 10% to almost nothing. I’ll wait.
Oh, there’s more? Sure is. It’s not like the power requirements of training models aren’t really obvious to the second most valuable company in the world, NVIDIA, with a current market capitalization of about US$3.2 trillion, second only to Microsoft. Why does NVIDIA come into this? Weren’t we talking about OpenAI? Well, NVIDIA provides the graphical processor units (GPUs) that all of that model training and execution runs on. Its biggest customers have been asking for faster AI compute for less power.
Enter Blackwell, NVIDIA’s latest GPU architecture. Why is it important for this? Is it because it’s twice as fast for training models and even faster for executing queries against them? No, although it is, it’s because it’s 25 times more energy efficient for training and queries. And yes, that does answer the question about grids that are dirtier and companies that aren’t Microsoft, for people wondering.
Go back to all the numbers that amounted to less than a gram per image or query and divide the grams by 25. Then please stop bothering me with expressions of outrage about that aspect of my use of power tools for research and image generation. People concerned about copyright and the jobs of creatives, please feel free to continue to fret, but I at least respect your concerns and am willing to have a discussion about them.
As a bonus, I’ll answer a question some may have had when I pointed to Tesla’s autonomous features about what relevance they have to this discussion. Teslas have big machine learning models running on custom GPUs running at absurd speeds integrating all of the sensor data flowing into them every second. If machine learning queries were the incredible power hogs that the current hysteria suggests, Teslas would be consuming more energy to run its autonomous features than to push the 2,800-kilogram car along highway at 110 kilometers per hour. Its battery would be twice or three times as big. And anyone who has ever written anything about both Tesla’s autonomous features and the horrific energy drain of machine learning models should have been able to connect the dots.
Have a tip for CleanTechnica? Want to advertise? Want to suggest a guest for our CleanTech Talk podcast? Contact us here.
Latest CleanTechnica.TV Videos
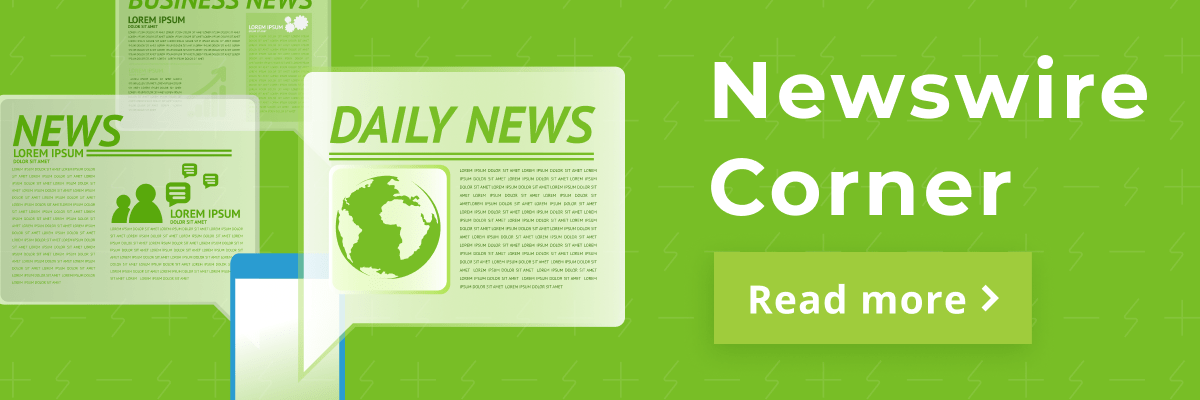
CleanTechnica uses affiliate links. See our policy here.